le titre du livre — 4. Data and systems
4
Data and systems
A brand built on innovation
Ahmed Hindawi, our founder, set out with a desire to do things differently from other publishers. The company understood the difficulties that publishers face when working across multiple systems. As a result, they began working on a system that would offer a full suite of end-to-end publishing services on one platform, specifically designed for the open access publishing model. This process gave the company experience in software development and ingrained a culture of innovation and agility that still thrives today. The eventual output of this end-to-end project was a platform called Phenom.
The arrival of Phenom
After a great deal of development, the first iteration of Phenom was released as an open source, end-to-end platform that allowed publishers to facilitate the entire submission-to-peer-review-to-publication process on one platform.
Its components were modulated, allowing publishers to customize the extent to which Phenom handled the end-to-end process. Since the first iteration, this modulation has also been of benefit to developers, as component parts can be developed independently of one another — a process that continues today.
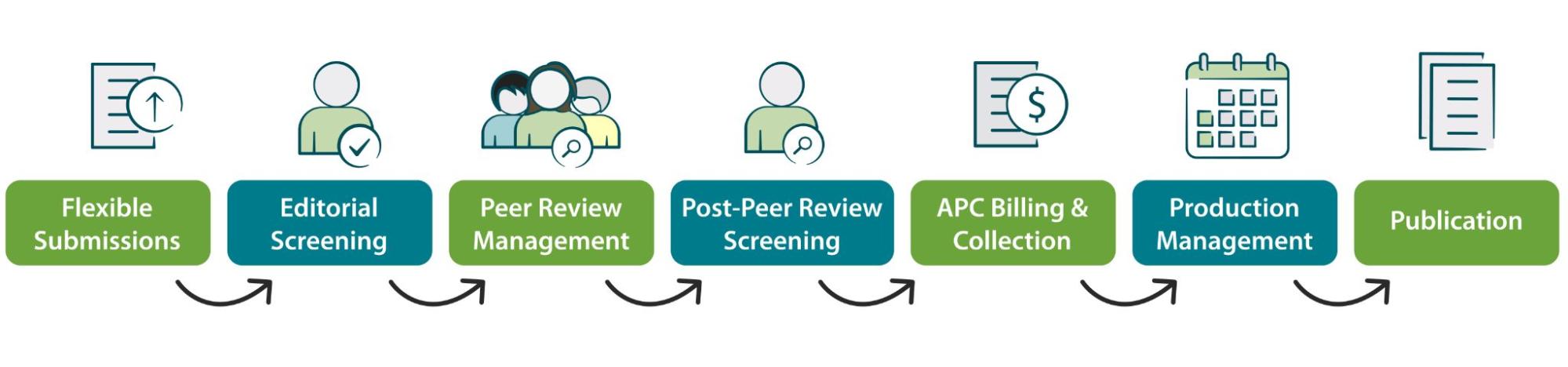
As we migrated our journals over to Phenom, another striking benefit of the system became clear: consolidated data. All actions on Phenom create ‘events’ that can be reported in one place. This centralization of end-to-end publishing data is a key enabler of many of the data systems that have allowed Journal Development to thrive.
Data-driven Journal Development
The availability of Phenom data was a significant driving factor in the development of data systems for Journal Development (JDev). Teams had access to real-time information on author, editor, and reviewer activity — providing the potential for much more relevant and timely communications with researchers, something the industry has traditionally struggled with.
However, the data needed to be professionally cleaned, handled, and processed for a marketing use case. Although initial efforts were made to do this through off-the-shelf offerings, it quickly became apparent that Journal Development would need dedicated data and marketing technology support to gain the most insight into, and fully utilize, the data they had.
Journal Development Data & Ops team
Following the demand for a dedicated technical resource, the Journal
Development Data and Operations (Data & Ops) team was formed to support
internal stakeholders.
Operational ethos
From the beginning, the team decided that operationally it was important to establish a foundational ethos on which systems could be developed. This can be broken into the following points:
-
Excellence at delivery
Ensure all platforms and campaigns make the best use of the technology available and meet the objectives of stakeholders -
Responsible and relevant use of user data
Protect and enhance user data where possible to create the best solutions for use cases, while still adhering to user permissions - Development agility
Create and maintain a dedicated development environment, allowing fast prototyping and innovation where required
Long-term aims
- Be the best Data and Marketing Technology resource in the industry
Be more agile, smarter, and compliant than any other operationally focused data team amongst the brand’s competitors
- Empower the researcher
Using data from our analysis, cycle insight back to researchers allowing them to make better decisions about their publishing choices and further the mission of open science
-
Develop team members
Support the team to become future leaders in the space, advance their careers and advocate for the industry, encouraging other technical specialists to work in the space
The resulting team covers three core functions: Data Engineering, Reporting and Insight, and Marketing Operations.

Teams within Data & Ops
The team’s key stakeholder relationships are with the other specialist units within Journal Development. Typically, the stakeholder relationships involve consultancy, requests, and delivery of campaigns against a submitted brief.

Top-level approaches and systems
The team has established a variety of approaches and top-level system architectures that underpin day-to-day activity. The section below outlines these approaches and systems but remains neutral to the specific technology deployed at the time of writing. This is deliberate as we want to maintain a strong emphasis on portability and reduce dependency on any specific service platform.
What is our approach to data collection?
Data collection happens constantly across both first- and third-party platforms. Regardless of what data is being collected, these three basic principles apply:
- Collect what is possible
- Process what is relevant
- Action what is required and permissioned
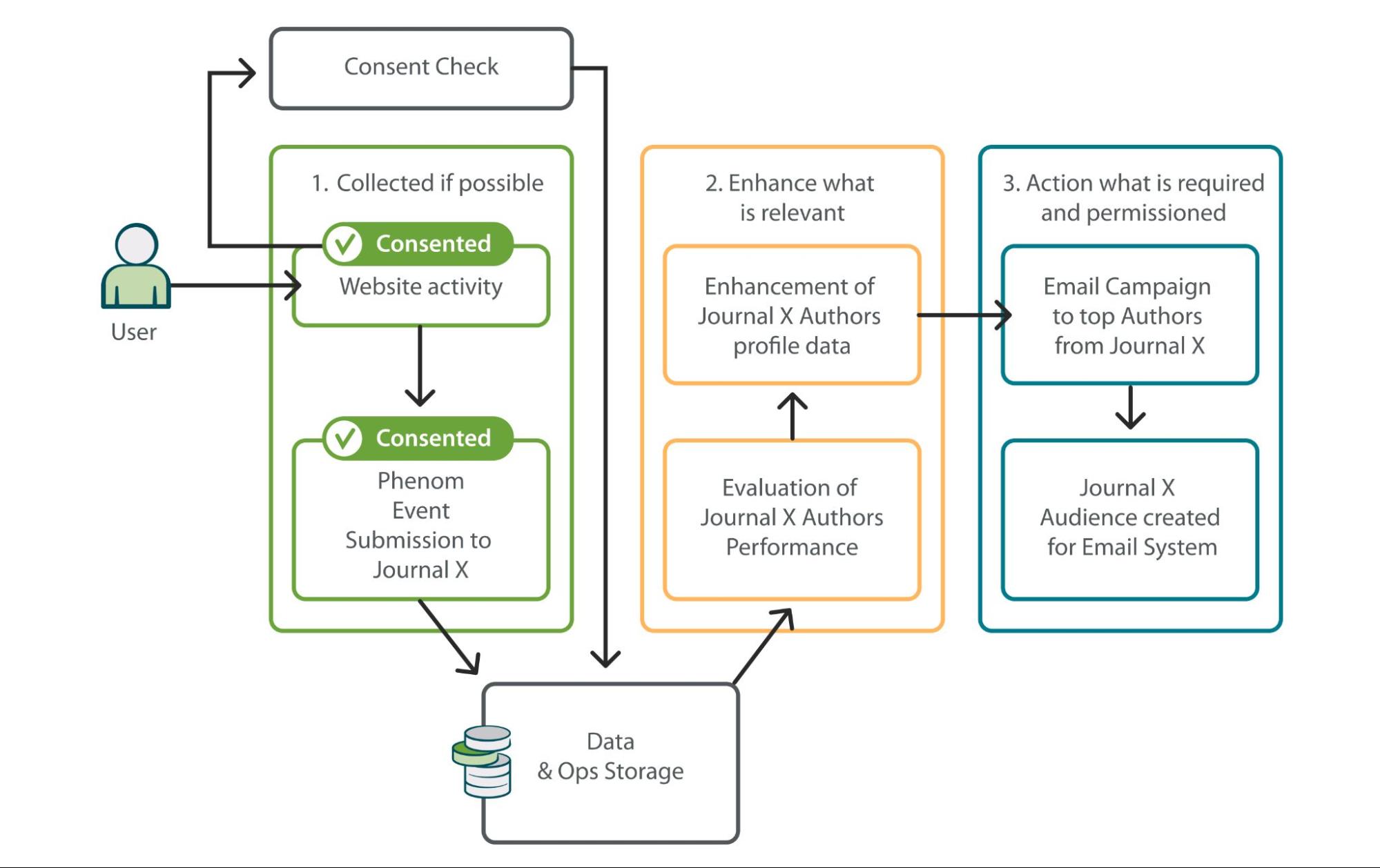
Core data objects collected
The objects below represent the main data objects the Journal Development Data & Ops team collect, process, and action to meet Journal Development requirements. There are other use cases where objects not on this list may also be ingested and processed for specific functions.
Researcher [user] data
- Collected through Phenom or through partnerships, where permissioned
- Enhanced in-house with data from Web Analytics and third-party vendors
Manuscript and journal data
- Collected through Phenom
- Enhanced in-house with data from third-party vendors
Event and behavioral data
- Collected through Web Analytics and Phenom
- Enhanced in-house with data from third-party vendors
What is our approach to augmentation?
Augmentation of data refers to
the improvement of the level of data surrounding a data object. For example, a basic user profile might include a name and an email
address. An augmented user profile might include a name, email, and career
history.
Where opportunities to augment data are present and are relevant to a use
case, the typical approach undertaken is to try and use industry-recognized
identifiers as key connectors between datasets. Where these are not available,
we look to create alternative linking methods to create commonalities between
datasets. This can involve amending data collection methods on platforms to
capture a metric or dimension that is known to exist in other datasets.

What is our approach to storage and syndication?
Storage and syndication are key considerations to how Data & Ops develop and distribute insights and audiences for stakeholders. Data Syndication is the process of sending data to other systems to deliver the same message across multiple systems. For example:
- An audience list is created in the database.
- It is then syndicated to an email system and social media for use in a campaign.
After a period of experimentation, it was established that the best option for the department would be to develop our own, customized database. This would allow for maximum flexibility to create data assets that could meet marketing use cases and could operate outside of the development lifecycle of other systems.
Storage and syndication through the Marketing Intelligence Database (MIDB)
The MIDB was created to be a central hub for Journal Development insight, while also allowing for a centralized place to store, process, and segment audience data to MarTech platforms.

The database employs an off-the-shelf, cloud-based data warehouse product and set of automated data pipelines to process, collect, and store a variety of first- and third-party data.
Once validated, logged and permission-checked, segmented audiences can be syndicated via a virtual computer to various AdTech platforms.
The MIDB also offers analysts and colleagues a platform to do exploratory analysis on existing datasets, delivering fast computing and automated query scheduling for dataset readiness. This has become particularly useful for ongoing, customized reporting and large-scale augmentation of data.
What is our approach to compliance and governance?
Our approach to both data governance and data compliance is ever-evolving. However, there are some core and consistent principles that we adhere to.
Processing
- Our basis for processing data is primarily Legitimate Interest and as such our approach to data management is always focused on ensuring this basis is being met
Governance
- Data security through quality assurance and adhering to administration by the principle of least privilege
- Regular review and development of processes
Compliance
- Compliance by design in system architecture
- Regular review and development of requirements stipulated by various data laws
- Systematically respond to all forms of requests from the Information Commissioner's Office
Common sense
- Develop a culture of ‘data for good purposes’ among system engineers
What is our approach to reporting?
Our top-level approach to reporting is to ensure all data are consolidated, cleaned, and presented in a table format within the MIDB. This is then connected to a front-end visualization platform to create an automated, single data source feed of insights for stakeholders.
Reporting can cover anything in Journal Development: from the presentation of campaign results, right through to market analysis and opportunity exploration. As such, off-the-shelf data warehouse solutions from cloud providers are excellent for this purpose. Fast data processing allows for speedy dashboards and a comprehensive development environment for analysts.
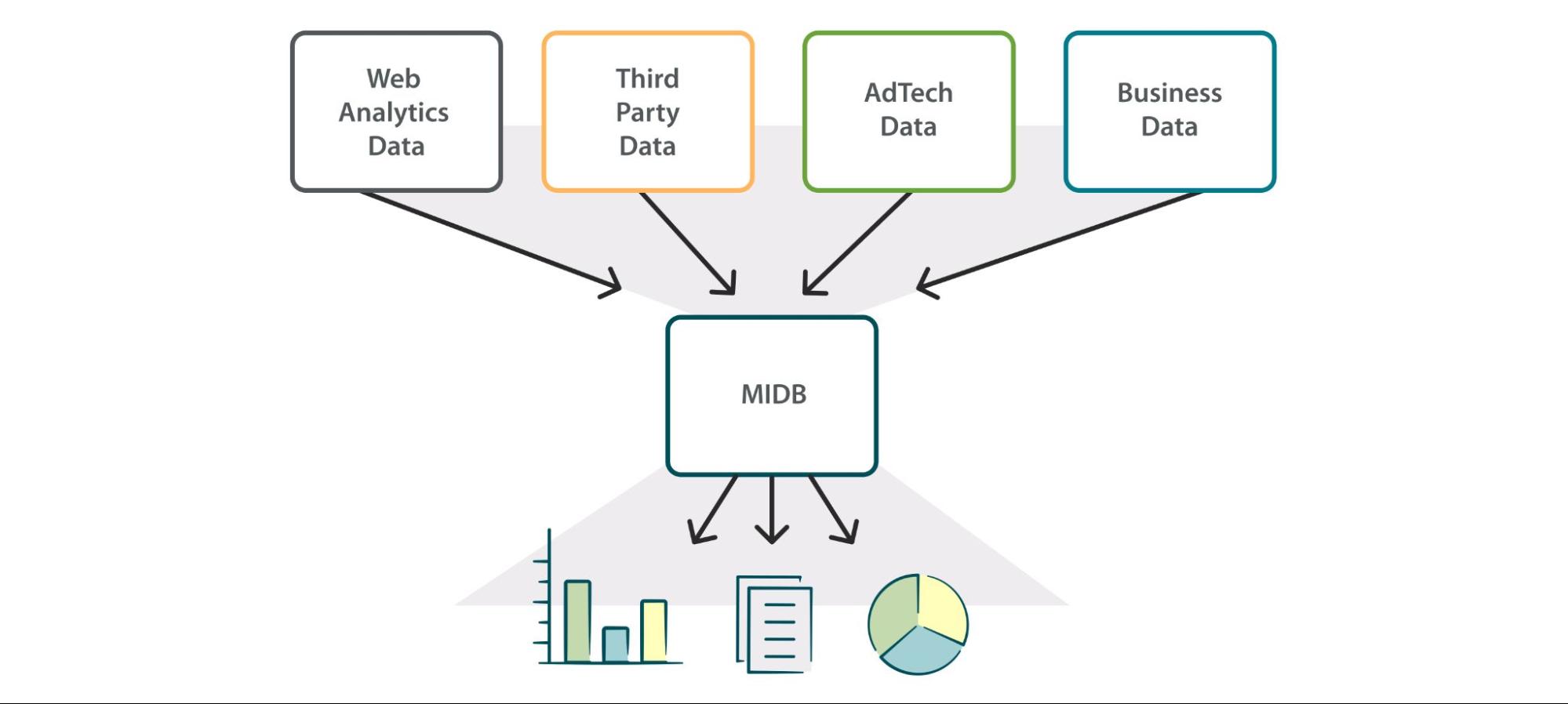
Attribution of Article Processing Charges (APCs) to marketing campaigns
Attributing APC value back to campaign spending is traditionally a difficult task. The peer review process can take many months to complete, meaning the value of conversions can remain unknown for a long time. On top of this, it is hard to ascertain a standard average order value, as APCs vary from journal to journal.
We have leveraged the MIDB to combine multiple datasets and build a picture of what activity happens in the lead-up to all APC payments.
The attribution method devised works by capturing data from each step in the process and working backward from the point of conversion to stitch interactions together. The process is as follows:
- Identify conversion within Phenom
- Pair the owner in Phenom with the owner’s web analytics data
- Search web analytics data for evidence of owner’s interactions with marketing campaigns
- Attribute a proportional value of the manuscript in Phenom to the channels that contributed to it

The data then outputs to a clean attribution table in the MIDB, updating daily as manuscript statuses progress through peer review.
Although we continue to develop this process, the principle of working backward from the point of conversion will remain central going forward.
Marketing Operations
The Marketing Operations team was formed in January 2022, bringing together the expertise of our communication platforms: email, paid, and social media. Designed to be a center of excellence, the team applies a thorough, expansive, and proactive approach to all marketing activities. This, in turn, improves processes, optimizes best practices for our ever-expanding programs, and drives greater engagement with our customers.
Our primary functions are:
- managing and optimizing the marketing technology stacks and platforms
- automating data processes
- facilitating marketing campaigns in the most effective, efficient, and compliant manner
- advocating a data-driven approach to audience profiling and segmentation
- developing new capabilities to enhance engagement
- tracking and analyzing performance data
Approach to campaigns
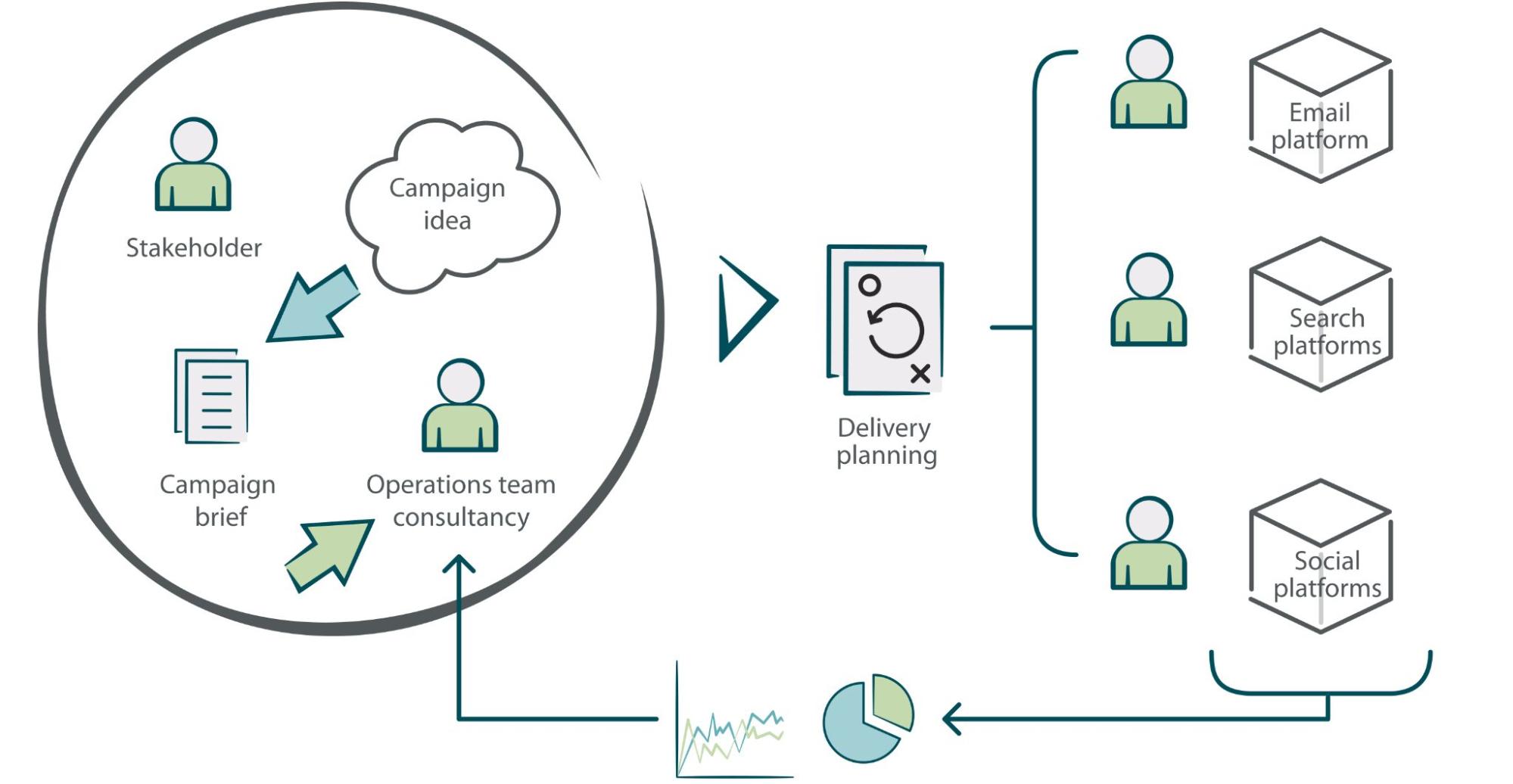
The campaign strategy — including its goals, messaging, and creativity — is defined by the individual marketing teams based on team, JDev, and wider business KPIs. We work closely with each team, offering a consultancy-like service to optimize campaign requirements and understand desired outcomes. We advise on:
- journey framework and building — incorporating channels, touchpoints, communication frequency, engagement splitting, predictive analytics, and much more
- A/B test and learn tactics
- dynamic content and personalization
- reporting methods
We encourage regular analysis of campaign performance with the idea to continually test > learn > re-iterate to gain a solid understanding of each customer segment and their behavioral trends. Promoting an insights-to-action approach enables us to constantly evolve, ensuring that communications remain relevant and we avoid oversaturating a particular customer segment.
Post-campaign launch, automated reporting provides stakeholders with top-level performance metrics for tracking and evaluation. This data is used to draw insights that can inform subsequent marketing and engagement activity. With the support of our analysts, we aim to report beyond open and click rates and instead tie APC spend back to each campaign and the marketing channels used. We consider this to be the ultimate indicator of success for each campaign.
Drive to automation
In 2021, we developed and initiated a plan to transform our email marketing platform. The need to automate data processes and campaign execution was the core driver of this transformation. The primary goal, aside from improving inefficiencies, was to remove all manual efforts marketers were previously undertaking. The plan focused on several areas for optimization:
- data governance and hygiene
- data infrastructure and architecture
- data processing
- automation
- marketing execution and effectiveness
- process change
- stakeholder and user education
- application of in-built predictive analytics
The most significant transformation came in 2022: the MIDB, an in-house built platform that centralizes data points from multiple internal data systems and platforms, was ingested into the email system and fed multiple layers of metadata. This completely revolutionized our data offering, and now provides us with a new and much-improved set of demographic, journal, manuscript, and customer-level information. This new infrastructure benefits us by:
- evolving our marketing approach across all communication platforms
- creating more sophisticated customer segments and profiles
- providing action- and behavioral-based segmentation
- offering more customer personalization
- providing tighter data governance
- synchronizing data across company platforms for greater consistency
- exchanging data across platforms for a multi-channel approach
Along with the MIDB, we also have a Customer Relationship Management (CRM) system which is synchronized to the email marketing platform as an added data source.

Email System Data Flow
Paid Media activity
Paid and social media platforms offer alternative marketing channels and are used for lead generation, raising brand awareness, journal promotion, and driving submissions. We proactively run tests across search engines and media advertising to monitor audience reach and engagement and determine which platforms have greater success at a campaign and individual level. Behavioral data from ad campaigns are passed on and tried on other ad channels as a method of remarketing. Similarly, tracking data is captured, ingested into the MIDB, and then made available in the email system.

Campaign delivery
So, we have optimized platforms, established a continually evolving process for our data sources, and now have a wealth of ever-expanding data points on offer for our marketers — how is this then leveraged to the optimum level?
Where what were once isolated campaigns now fall into a wider customer journey, the concept of creating automated journeys is instead beginning to be at the forefront of marketers’ minds. Examples of this are welcome/onboarding and author submission journeys which provide a streamlined and timely set of relevant communications based on customer action. Not only does this improve the overall customer experience but it has also increased marketing teams’ capacity, enabling them to focus more time on campaign planning.
Whilst automated journeys are continuously running at the required schedule, the majority of campaign delivery is in the form of one-off activities via the desired channel.
The increased data points have also resulted in a greater opportunity for communications to be further optimized by including personalization and/or dynamic content at either contact or journal/manuscript level so a single template can serve multiple segments and be specific to their particular history.
System-defined predictive analytics is added to all journeys which results in email messages being deployed at the time the individual is likely to interact.
Here is where the multi-channel approach comes into play. Marketers are planning with a wider lens so all touchpoints relating to a single campaign are considered as part of a journey. In this instance, there are opportunities to segment audiences and assign them to multi-platforms before launch, for example, email disengagers to be served content via media channels. Likewise, this method can be applied mid-journey where customers who did/did not interact with email campaigns receive media touchpoints and vice versa.

Platforms managed by Data & Ops
The list below reflects the technology in use at the time of writing this documentation. As mentioned in previous sections, we intend to maintain flexibility over which platforms we use, reducing dependencies where possible.
Advertising Platforms
- Google Search Ads
- Bing Search Ads
- Twitter Ads
- Facebook Ads
Analytics Platforms
- Google Analytics
- Google Search Console
Email / CRM platforms
- Salesforce CRM
- Salesforce Marketing Cloud
- Dotmailer
Infrastructure / Data Technical
- Asana Ticketing System
- Team GitHub Repository
- Team Confluence Library
- Google Cloud Platform
- Google BigQuery
- Google Cloud Storage
- Google Tag Manager
- Funnel.io
- Hotjar
- GetSiteControl
- Secure File Transfer Protocol (SFTP)
Data & Ops Dependencies
We have two critical dependencies, one where we are the stakeholder and the other where we are the service provider.
Dependency on Phenom Databases
Our core data tables rely on regular updates from Phenom’s reporting databases. If for any reason those databases are unavailable or make a change that impacts the insight we are providing, we see the impact immediately. To mitigate against this, our systems are built to read automatically but retain the ability to manually refresh if needed. This guards against outages caused by database downtime during a scheduled refresh. The team also maintains strong working relationships with various data teams around the business, to ensure we have access to all available insight.
Supplier dependency on wider Journal Development team
Data & Ops supply data to the various engagement teams across Journal Development. We have an outfacing dependency on them as this data supports their campaign activity and drives their objectives. Any breakdown in supporting pipelines is detected within an hour due to round-the-clock monitoring. Issues are then worked on until fixed under high priority.